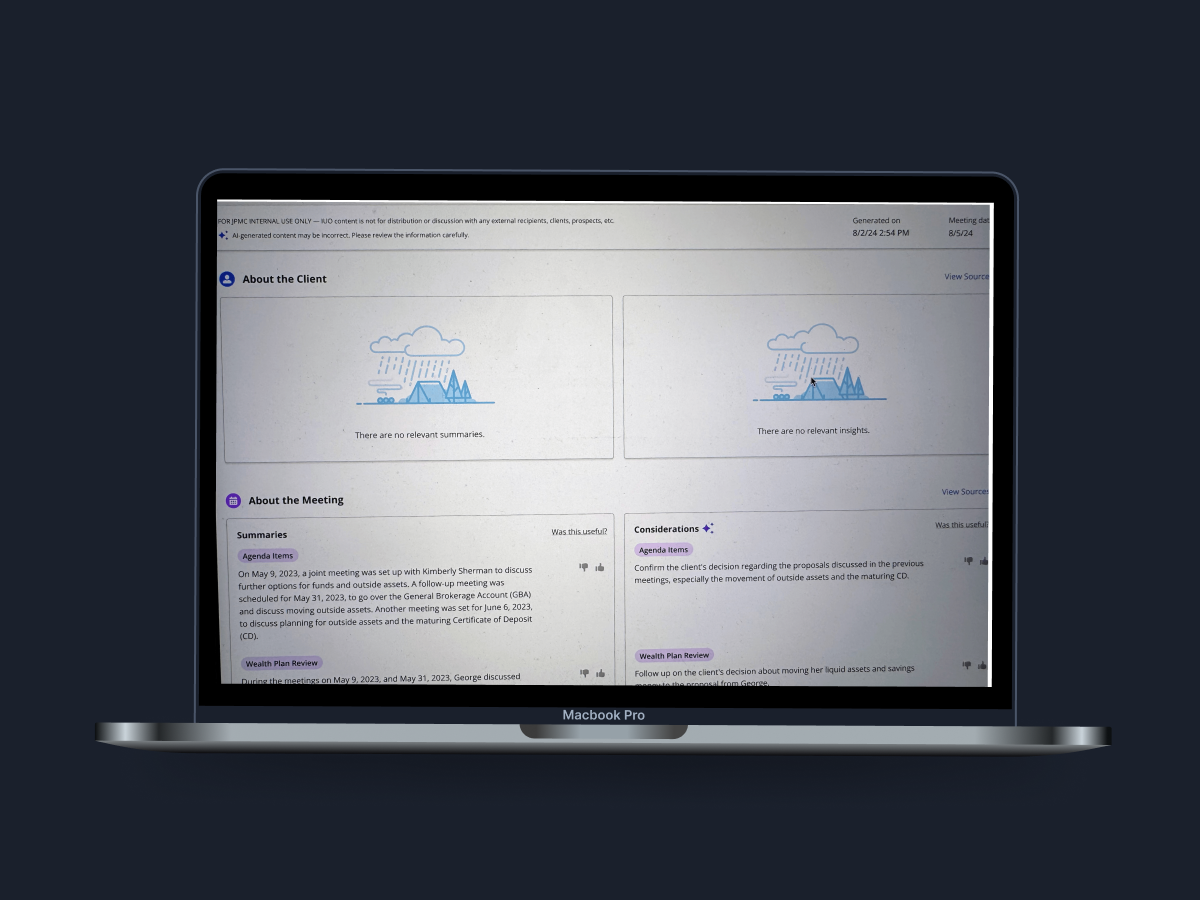
Introduction
Meeting Assist is a generative AI tool that will be used to help Private Client Advisors in the Wealth Management division at JP Morgan Chase prep for their quarterly meetings with their clients.
Timeline
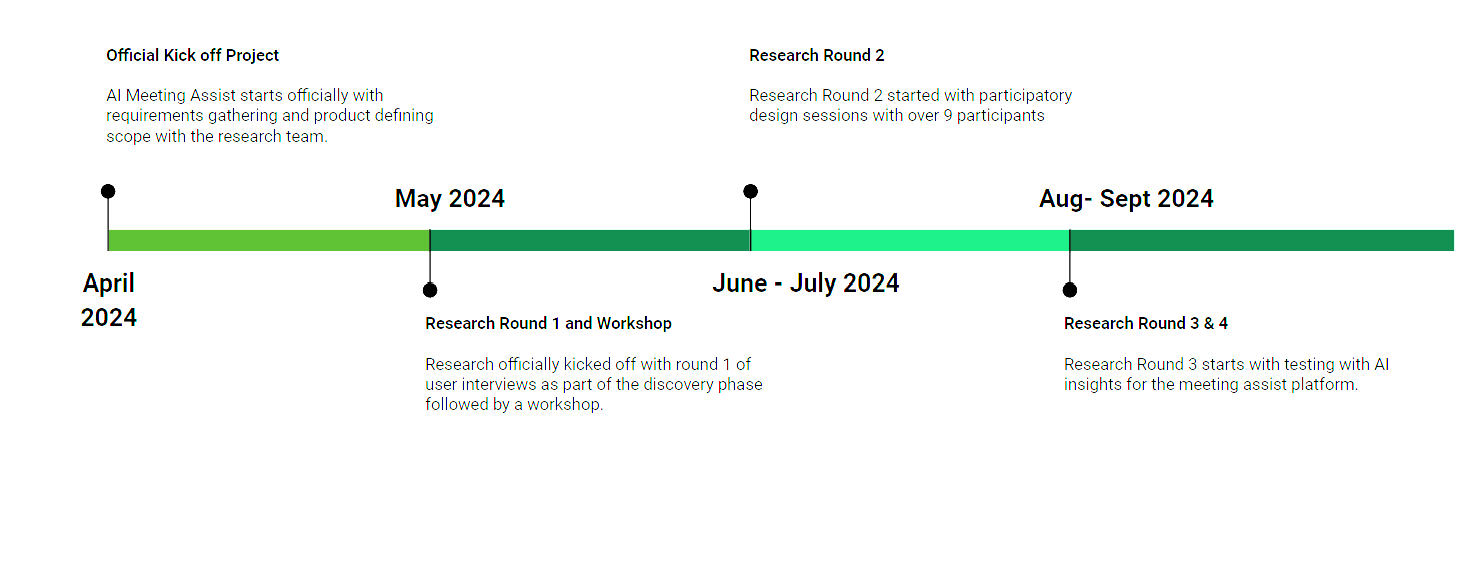
Goals and KPIs
• The goal of the overall project was to make the insights of AI overall useful.
• 75% Increased engagement and utilization of Meeting Assist by advisors by 2025.
• KPIs used during this project were:
⁃ Overall satisfaction with the interface,
⁃ NPS score (this user metric will be used in future rounds of research),
⁃The usefulness of AI insights will improve by 25 percent end of the year and 75 percent by next year (2025).
Time constraints were a challenge
• The AI space is relatively new so there was a lot of dependencies and unknowns on whether the AI insights would actually be useful for our target audience.
• Time constraints for this project were a huge barrier to timely results and analysis.
• Collaboration between the design, product, machine learning team, and content teams was very crucial to helping the research tests be successful.
• MVP was set for October to November so research efforts had to ramp up before October.
Who was our Audience?
• The focus of this project was to recruit internal participants as a way to test out the usefulness of AI insights before moving it into production with chase clients (belonging to the wealth management product)
• Our target audience included primarily Private Client Advisors along with Private Client Investment Advisors (almost like assistants to Private Client Advisors)
Collaboration Tools used in the project
• Figjam & Figma were primarily used during the requirements gathering phase and helped the research and design team generate ideas together.
• Figma was primarily used not only in the participatory design test but also in the user/insights test.
• Primarily the research team used Zoom and Figma to conduct the different research rounds.
Discovery Phase: User Interviews
User interviews were helpful in creating a baseline for how Private Client Advisors work with their clients, who their clients were
I collected qualitative responses from the user interviews through a topline report to understand how our personas work and how AI would or could eventually work for them (the caveat being we didn’t say AI would be involved in the future)
Quotes by the users:
“If AI could help me enhance my workflow, this could be useful to me”
“Usually clients trust me when we know about their personal lives as they trust me with their money. So this needs to give me tailor-recommended insights”
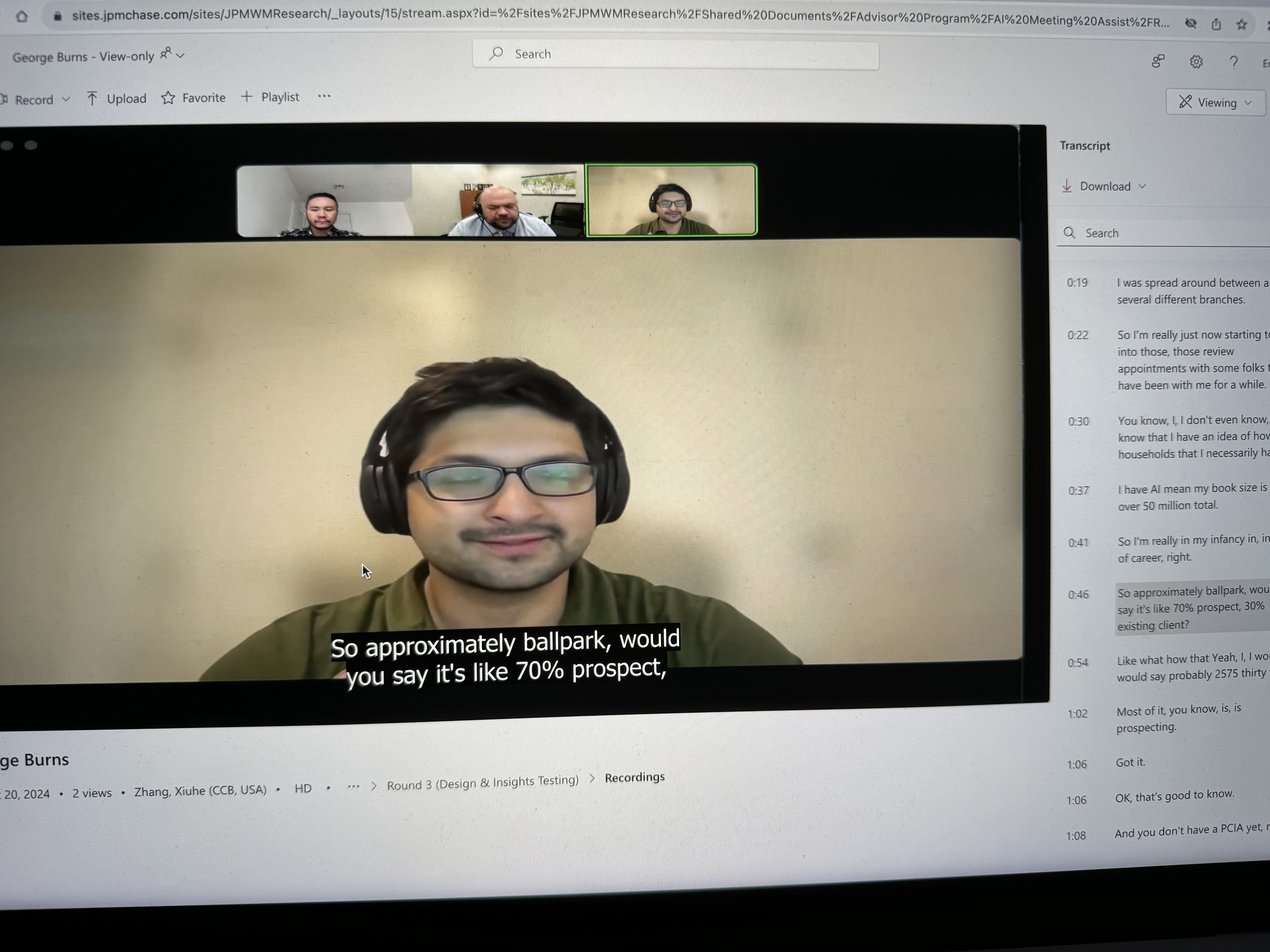
The Workshop was a bridge between Round 1 & 2 research
I led the research team workshop where we tried to gather feedback from different internal stakeholders
The research team wanted to validate the four different sections and what advisors would like to see in there to test out the content and where the AI and how the AI could help the advisors.
We took these insights and created a participatory study to test this out with our advisors.
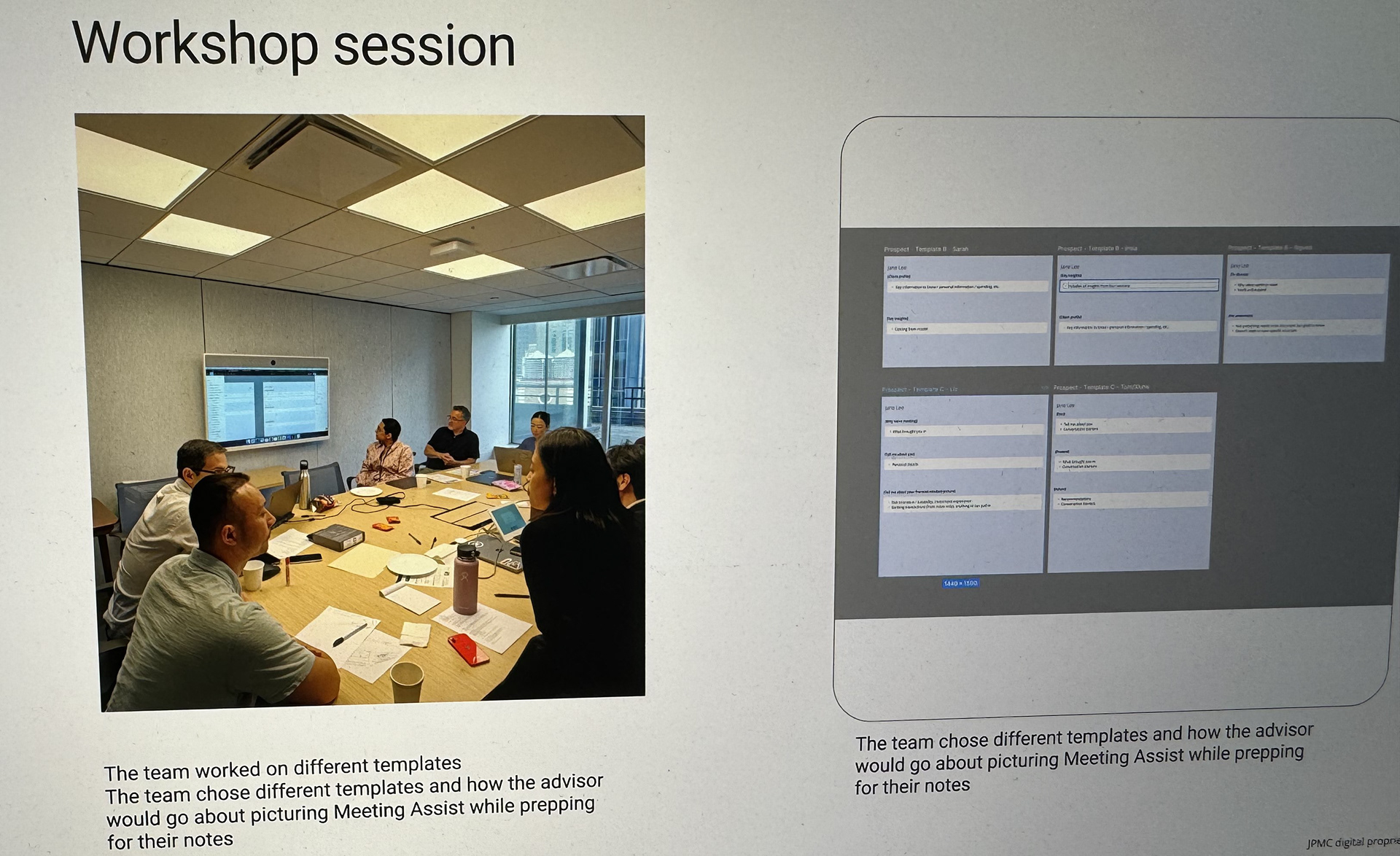
Why did we use Participatory Design?
After the Research round 1 and the workshop, we came to a conclusion that advisors had four mindsets while visualizing their Meeting Assist tool namely ‘Tell me about yourself, Reason for Meeting, Financial Mindset and Financial Recommendations’.
We decided to conduct a Participatory Design is a study to test out where the different sections could be and the labels of content and we waited on approval to conduct insights testing.
We had dry runs with internal teams like Product Managers, Content designers, and other team members to help us time this study as we weren’t sure if we would have enough time to complete the study given the questions to be answered
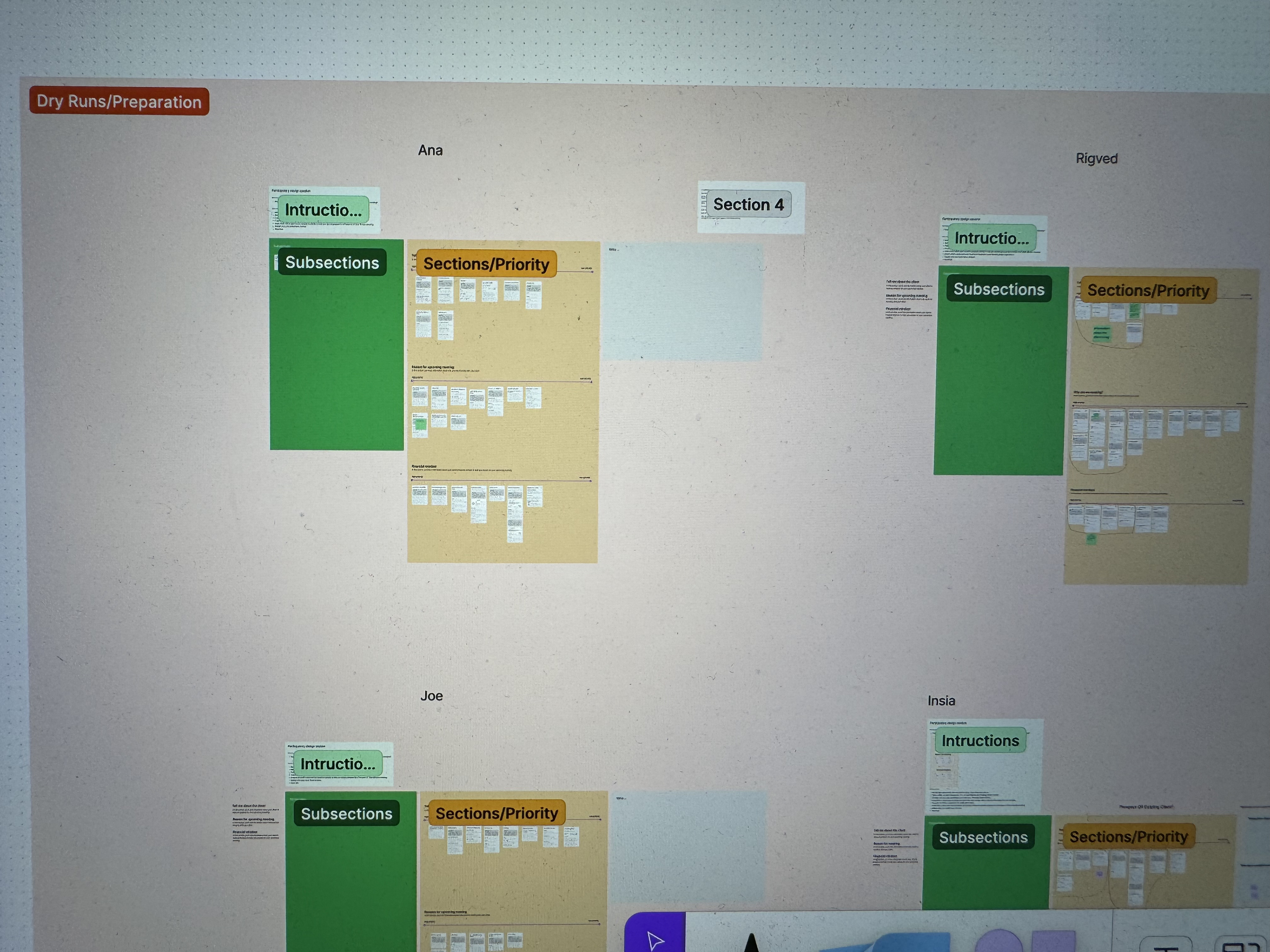
What did we find out?
• From the Participatory Design study we found that our hypothesis was valid and the four mindsets are what advisors did indeed want.
• We also found out that advisors would not want a mobile form of Meeting Assist as their meetings were usually conducted in Chase branches and they preferred to have other applications like Advisor Central as well in the background at least until Meeting Assist’s AI insights get better.
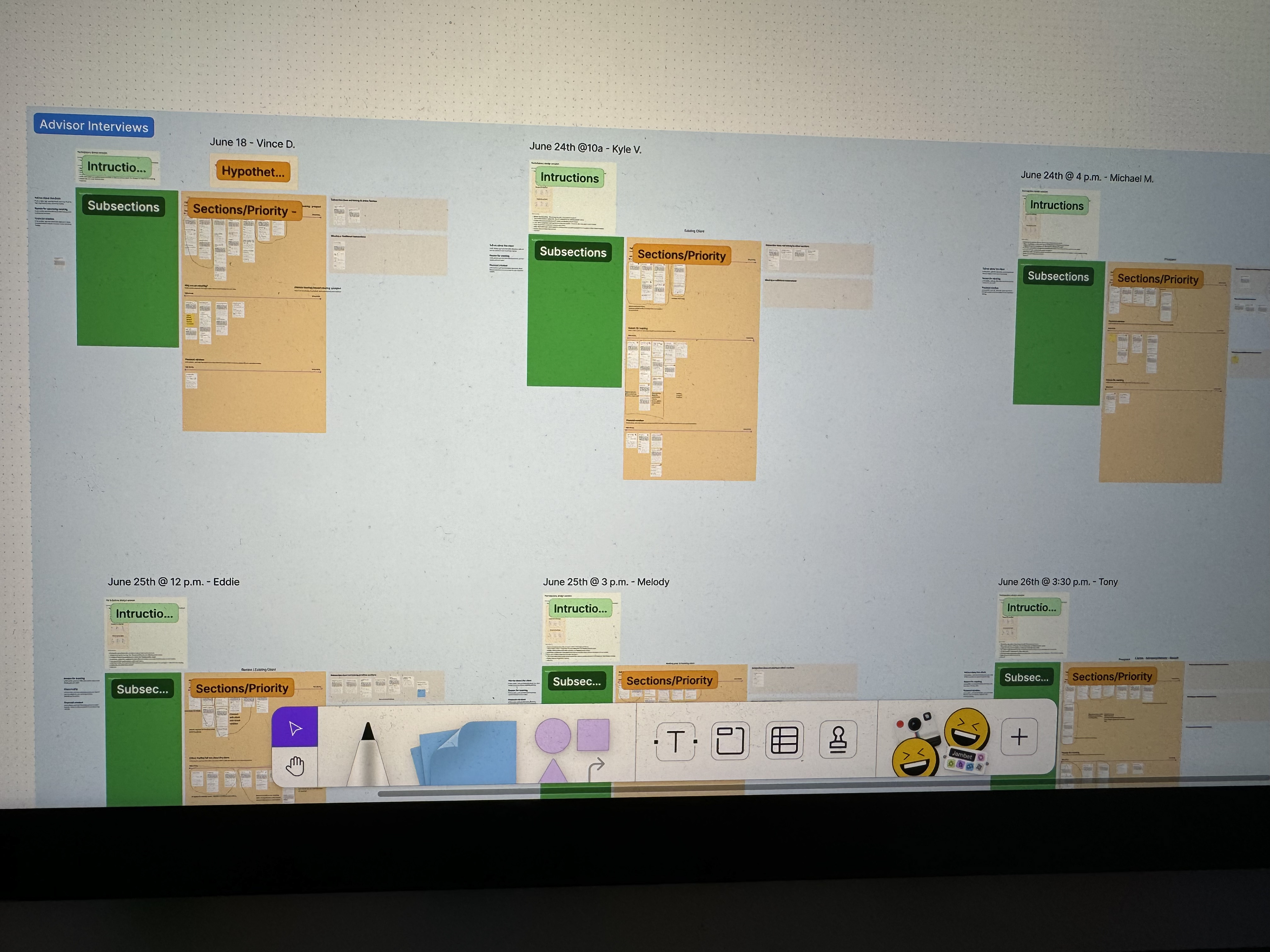
User/Insights testing
The goal of the Usability/Insights test was to understand the usefulness of the AI insights, organizational structure, content, and the entry point to how advisors would visualize meeting assist could be from.
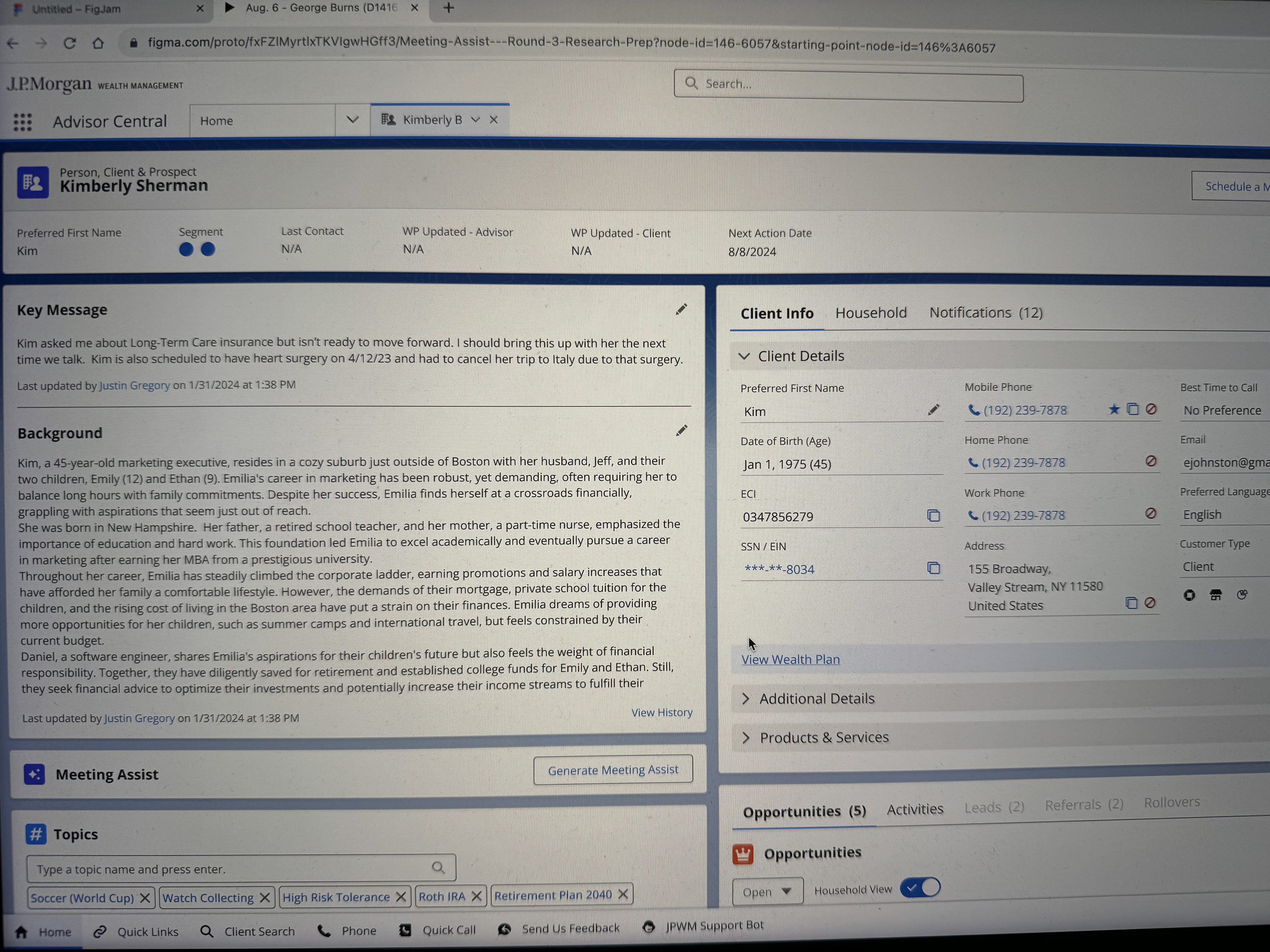
• From the insights we found that advisors really needed the AI to be more useful in their recommendations.
• Advisors also didn’t like the placeholder design for sections that didn’t have content.
• Overall the usefulness of AI insights and Summaries needs to be refined which would help the Machine Learning team tailor-made the insights to what advisors said in the test.
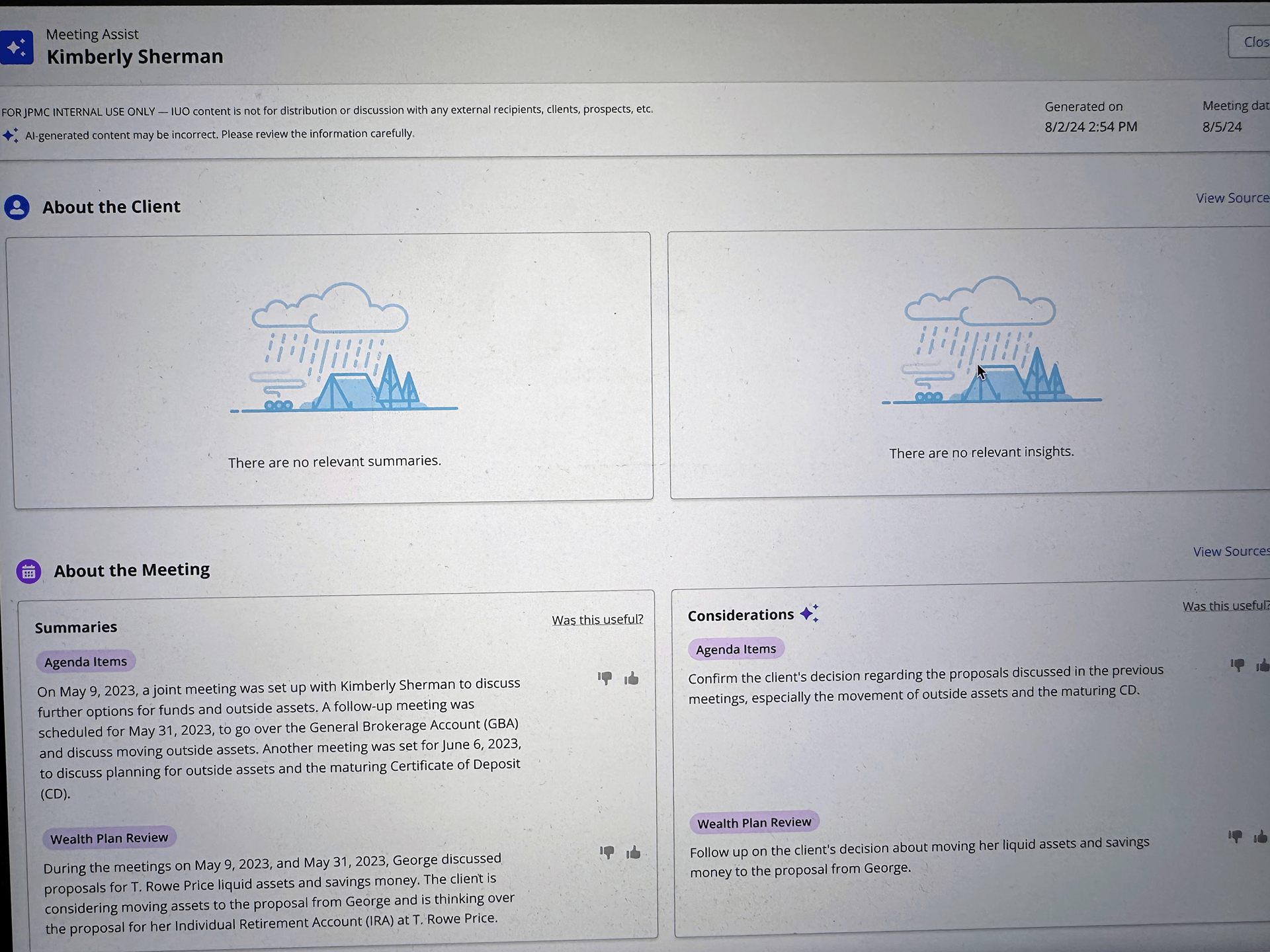
Concept Test helped improve overall design look
• We were also working on creating new concepts (all the wireframes were designed by me using the Salesforce design system) to test out different ways of how AI could be showcased that would actually be useful for advisors. The wireframes were created in Figma
• The concept test would be in conjunction with the insights being tested which would help reduce our time spent in future research rounds testing the usability of these wireframes
• The goal is to make AI insights 25% more useful using qualitative feedback from every research round and using prompt engineering to improve AI insights to produce a net positive feedback overall before next year.
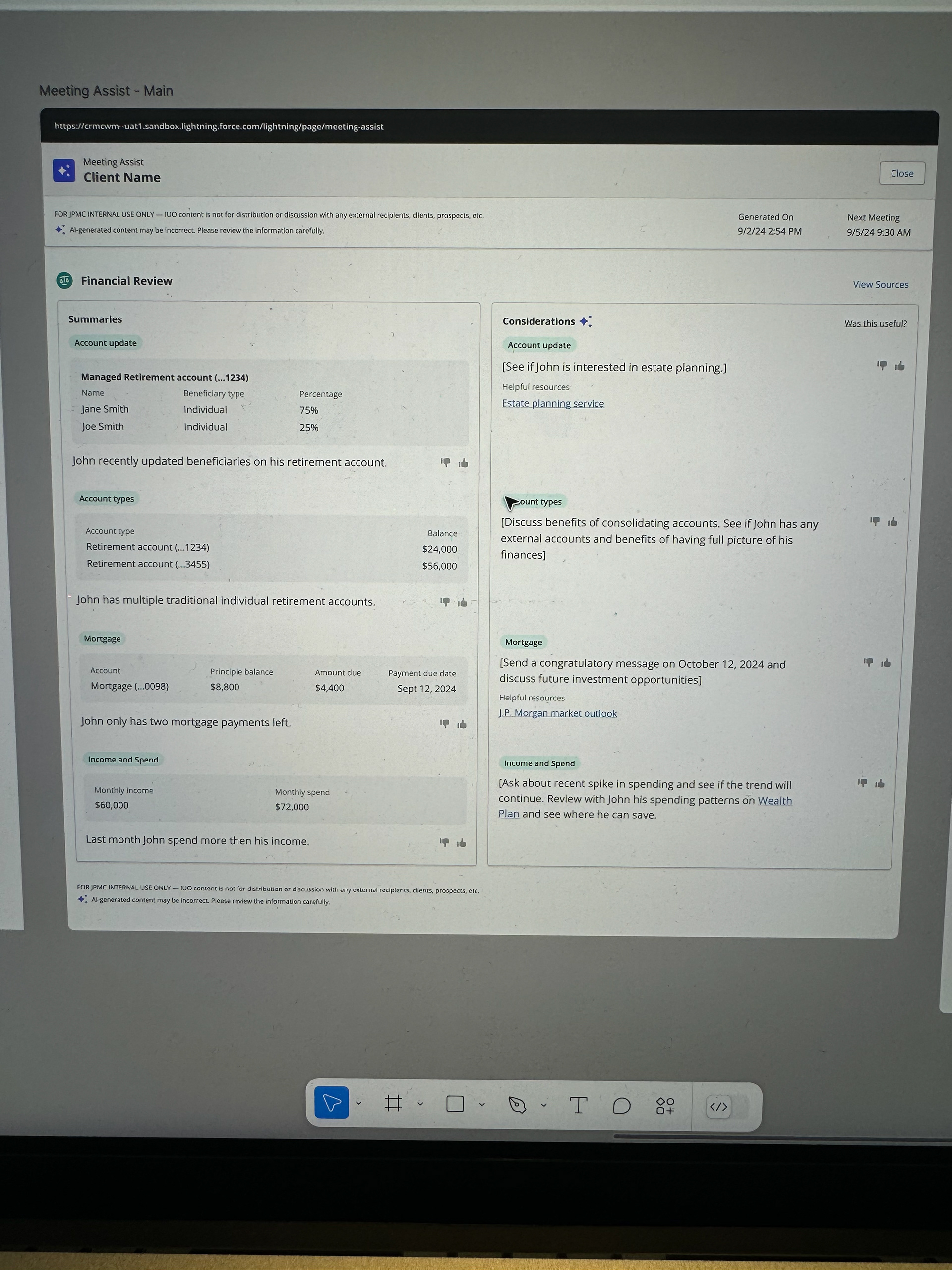
Another goal of the concept/insights test is to test out the blank-spaced sections as AI in the last round of research could not sometimes fill out certain sections as there wasn’t enough information about the clients.
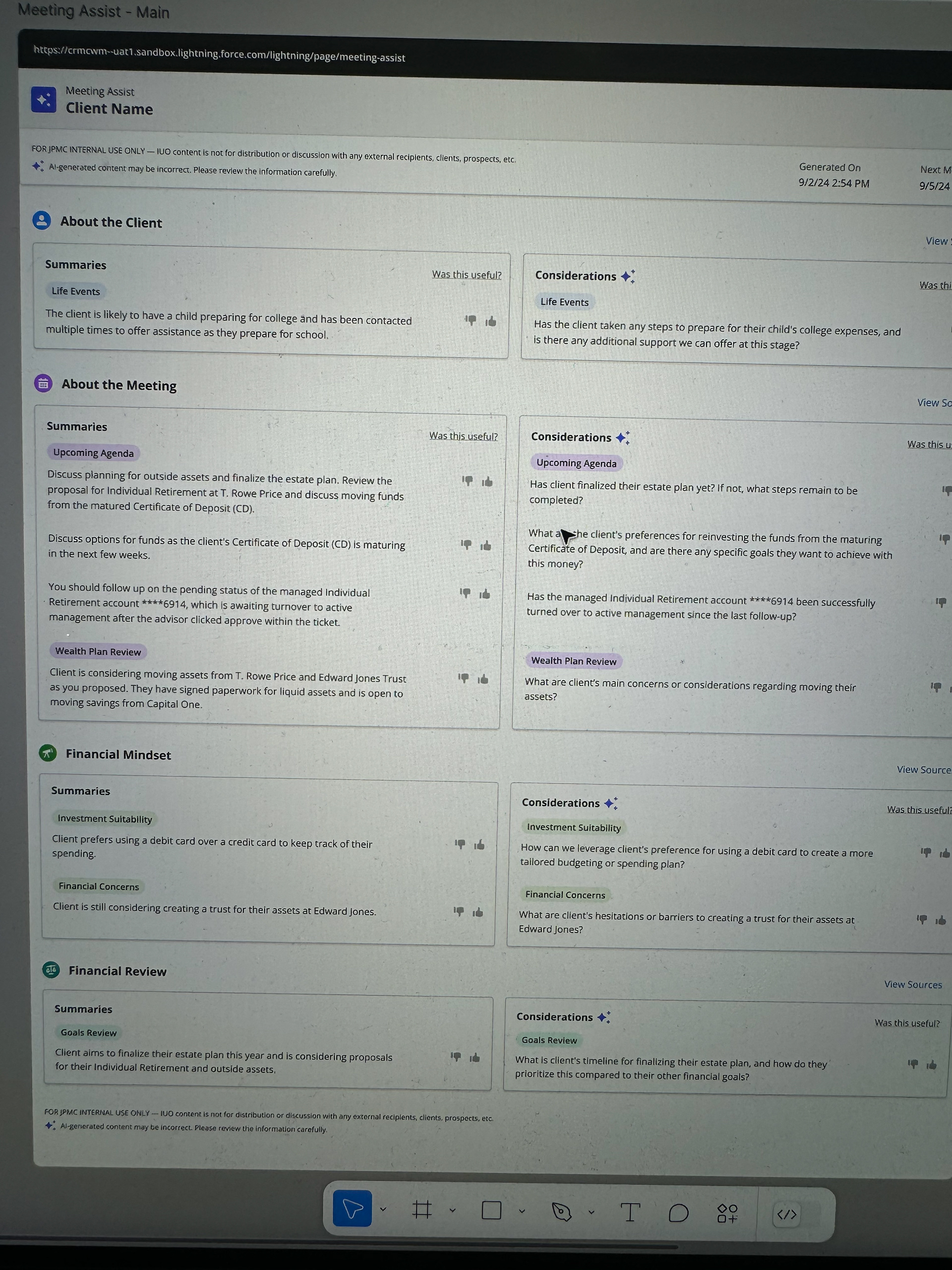
Conclusion
Currently, the research team is conducting Round 4 of interviews to test out the utility of AI insights after the Machine Learning and Content team did their Prompt engineering magic to the AI insights.
This iteration will help us understand whether the Meeting Assist tool will be useful for advisors. Currently, JPMC is going through an AI innovation phase but there is a lot of we will test it out with our internal advisors before showcasing it to our clients.
Future Things to Consider
Push leaders to test out AI ethics as we feed sensitive data into the Large Language Models. Regulatory bodies around a place like Chase seem far too relaxed with respect to privacy concerns about users.